A lockdown project
A couple of years ago, after evaluating the available open-source solutions, I became interested in the idea of writing a low-latency websocket server for the JVM. The project I was working on required a high-throughput, low-latency server to provide connectivity to a clustered backend service. We started by writing some benchmarks to see whether the most popular servers would be fast enough. In the end, we found that an existing library provided the performance required, so the project moved on. I still had the feeling that a cut-down and minimal implementation of the websocket protocol could provide better overall, and tail latencies than a generic solution.
The project in question was the Hydra platform, a product of Adaptive Financial Consulting[1], created to help accelerate development of their clients’ applications. The Hydra platform uses Aeron Cluster[2] for fault-tolerant, high-performance state replication, along with Artio[3] for FIX connectivity, and a Vert.x[4]-based websocket server for browser/API connectivity. Users of Hydra get a “batteries included” platform on which to deploy their business logic, with Hydra taking care of all the messaging, fault-tolerance, lifecycle, and state-management functions.
Vert-x was the right choice for the platform, as it provided good performance, and came with lots of other useful functionality such as long-poll fallback. However, I was still curious about whether it would be possible to create a better solution, performance-wise, just for the websocket connectivity component. During the UK’s lockdown period, I found time to begin development on a new project, of which Babl is the result.
High Performance Patterns
I have been working on low-latency systems of one sort or another for over 10 years. During that time the single-thread event-loop pattern has featured again and again. The consensus is that a busy-spinning thread is the best way to achieve low latencies. Conceptually, this makes sense, as we avoid the wake-up cost associated with some kind of signal to a waiting thread. In practice, depending on the deployment environment (such as a virtual cloud instance), busy-spinning can be detrimental to latency due to throttling effects. For this reason, it is usually reserved for bare-metal deployments, or cases where there are known to be no multi-tenanting issues.
Another well-known approach to achieve high performance is to exploit memory locality by using cache-friendly data-structures. In addition, object-reuse in a managed-runtime environment, such as the JVM, can help an application avoid long pause times due to garbage collection.
One other performance anti-pattern commonly seen is the usage of excess logging and monitoring. Babl exports metrics counters to shared memory, where they can be read from another process. Other metrics providers, such as JMX will cause allocation within the JVM, contributing to latency spikes. The shared memory approach means that a ‘side-car’ process can be launched, which is responsible for polling metrics and transmitting them to a backend store such as a time-series database.
By applying these techniques in a configuration inspired by Real Logic’s Artio FIX engine, I aimed to create a minimally functional websocket server that could be used in low-latency applications, such as cryptocurrency exchanges. The design allows for socket-processing work to be scaled out to the number of available CPUs, fully utilising the available resources on today’s multi-core machines.
Architecture
The Babl architecture separates the concern of socket- and protocol-handling from the needs of the application developer. The intent is that the developer needs only implement business logic, with Babl taking care of all protocol-level operations such as framing, heartbeats and connections.
Socket processing and session lifecycle is managed in the session container, instances of which can be scaled horizontally. The session container sends messages to the application container via an efficient IPC, provided by Aeron. In the application container, the user’s business logic is executed on a single thread, with outbound messages routed back to the originating session container.
The image below shows how the various components are related.
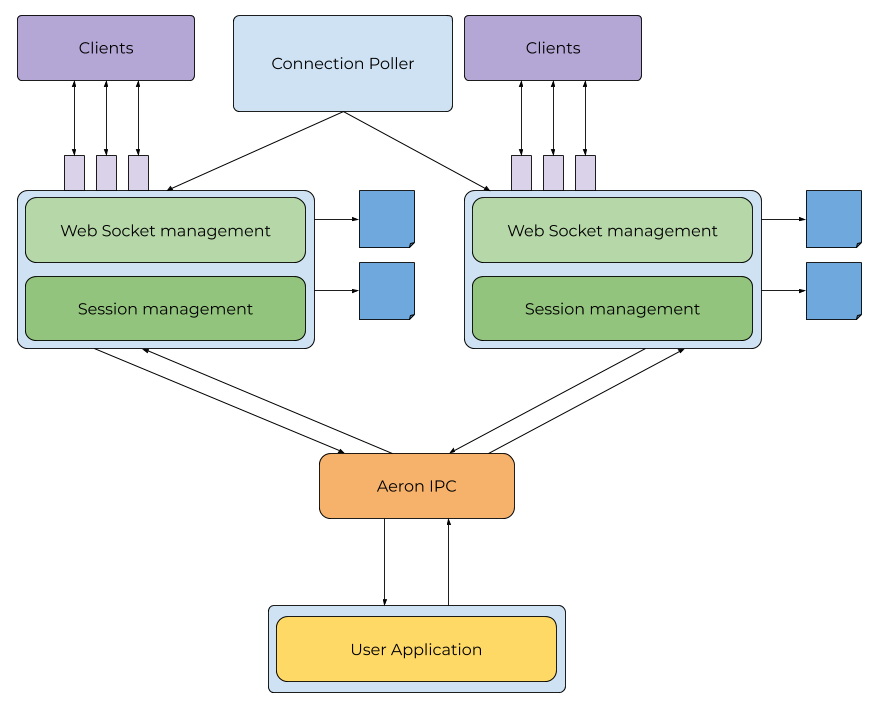
Use cases
Babl is designed for modern applications where latency is a differentiator, rather than as a general web-server. For this reason, there is no long-poll fallback for use in browsers that are unable to establish web-socket connections; nor is there any facility for serving web artifacts over HTTP.
If HTTP resources need to be served, the recommended approach is to use some static resource server (e.g. nginx) to handle browser requests, and to proxy websocket connections through the same endpoint. Currently, this must be done through the same domain; CORS/pre-flight checks will be added in a future release. An
example of this approach can be seen in the Babl github repository.
Configuration
Babl can be launched using its APIs, or via the command-line using properties configuration. The various
configuration options control everything from socket parameters, memory usage, and performance characteristics.
To quickly get started, add the Babl libraries to your application, implement the
Application interface, and launch Babl server from the
command-line or in a
docker container.
Performance
Babl has been designed from the beginning to offer the best available performance. Relevant components of the system have been benchmarked and profiled to make sure that they are optimised by the JVM.
JMH benchmarks demonstrate that Babl can process millions of websocket messages per-second, on a single thread:
Benchmark (ops/sec) Score Error
FrameDecoder.decodeMultipleFrames 13446695.952 ± 776489.255
FrameDecoder.decodeSingleFrame 53432264.716 ± 854846.712
FrameEncoder.encodeMultipleFrames 12328537.512 ± 425162.902
FrameEncoder.encodeSingleFrame 39470675.807 ± 2432772.310
WebSocketSession.processSingleMsg 15821571.760 ± 173051.962
Due to Babl’s design and tight control over threading, it is possible to use thread-affinity to run the processing threads (socket-process and application logic) on isolated cores to further reduce latency outliers caused by system jitter. In a comparative benchmark between Babl and another leading open-source solution, Babl has much lower tail latencies due to its allocation-free design, and low-jitter configuration.
In this test, both server implementations use a queue to pass incoming messages to a single-threaded application. In the case of Babl, this is Aeron IPC, for the other server I used the trusted Disruptor with a busy-spin wait strategy. The application logic echoes responses back to the socket processing component via a similar queue, as shown below:

Each load generator thread runs on its own isolated CPU, handling an even number of sessions; each session publishes a 200-byte payload to the server at a fixed rate, and records the RTT.
Both server and client processes are run using OpenOnload to bypass the kernel networking stack.
The load generator runs a 100 second warm-up period at the specified throughput, before running a 100 second measurement period; this ensures that any JIT compilation has had time to complete.
The work done on the server includes the following steps:
Socket read
Protocol decoding
Thread hop on inbound queue
Application logic (echo)
Thread hop on outbound queue
Protocol encoding
Socket write
Benchmark Results
Response times are expressed in microseconds.
10,000 messages per second, 1,000 sessions
| Min | 50% | 90% | 99% | 99.99% | Max |
Babl | 29.3 | 56.3 | 81.7 | 144.8 | 213.9 | 252.2 |
Vert.x | 33.3 | 70.0 | 106.8 | 148.0 | 422.4 | 2,013.2 |
100,000 messages per second, 1,000 sessions
| Min | 50% | 90% | 99% | 99.99% | Max |
Babl | 25.2 | 55.3 | 81.5 | 131.8 | 221.1 | 393.2 |
Vert.x | 34.2 | 73.8 | 95.9 | 132.7 | 422.4 | 10,002.4 |
Getting Babl
Babl’s
source-code is available on Github, and releases are published to
maven central and
docker hub.
The benchmarking harness used to compare relative performance is also on Github at
https://github.com/babl-ws/ws-harness.
Thanks
Special thanks to Adaptive for providing access to their performance testing lab for running the benchmarks.
Links
https://weareadaptive.com
https://github.com/real-logic/aeron
https://github.com/real-logic/artio
https://vertx.io/
Enjoyed this post? You might be interested in subscribing to the Four Steps Newsletter, a monthly newsletter covering techniques for writing faster, more performant software.